How player age (goal)impacts performance
How players age, The Relative Age Bias and why the "average" is not the Particular.
The Aging Curve
A huge advance in the Goalimpact algorithm was to investigate the average Impact of all players in dependence on their age. The result is the Goalimpact Aging Curve, which shows how, on average, a player's impact on the goal difference develops with age. This allows football managers to reliably predict how a player might perform in the future - a true game changer.
In this essay, we'll explore key findings related to aging in football and explain why I was “discriminated” due to my birthday.
Peak Performance and the golden player age
A key finding of the Aging Curve is that a "Best Player Age" exist. The graph shows that an average player reaches his Goalimpact Peak (highest impact on the goal difference) around 27. Such age is often discussed in the public discourse as the golden player age (german: "bestes Fußballeralter" = best football player age). A testimony to the wisdom of crowds effect.
We presume that at the age of 27 a player’s athletic abilities did not deteriorate yet, but game experience begins to pay off. Hence a combination of the two aspects results in the highest impact on the score.
Interestingly, a player's impact remains relatively stable over the next years. There is only a ~10% decrease between the age of 27 and 35. However, above 30, the deviation from the average probably increases. Why? We expect some players to decline less while others will strongly experience a Goalimpact collapse. What might cause such deviation? It is not “position” per se because the Goalimpact of defenders, midfielders, and strikers declines almost identically (except for goalkeepers, see below). However, there might be a crucial separating factor that determines how a player ages.
Sprinting - the aging separator?
Even though the Goalimpact aging factor does not depend on player position, it likely relies on the players’ skill portfolio. Especially sprinting performance decays to a great degree after a certain age. Thus we assume that fast players age more quickly. This hypothesis is backed by comparing the Olympic results for the men’s 100-meter sprint. An analysis of the winners between 1996 and 2016 shows that most gold medal winners are 26 years old. More, almost all medals go to sprinters between the ages of 23 and 30.
However, in football, rarely someone needs to sprint for 100 meters. Rather more relevant seem to be the first 10 meters. Thus, acceleration is more crucial but likely to be impacted by age. Hence, players who are extremely fast and impact to the game with sprinting probably have a more pronounced decline than players with different talents. (We will eventually test that hypothesis.)
Old but Gold Goalkeepers
Goalkeepers can demonstrate the most extreme example of the interdependence between skill and aging. Compared to field players, Goalkeepers have their highest Goalimpact at age 33, a + 6-year difference. Furthermore, the decline is shifted toward older ages. Both differences require some reasoning.
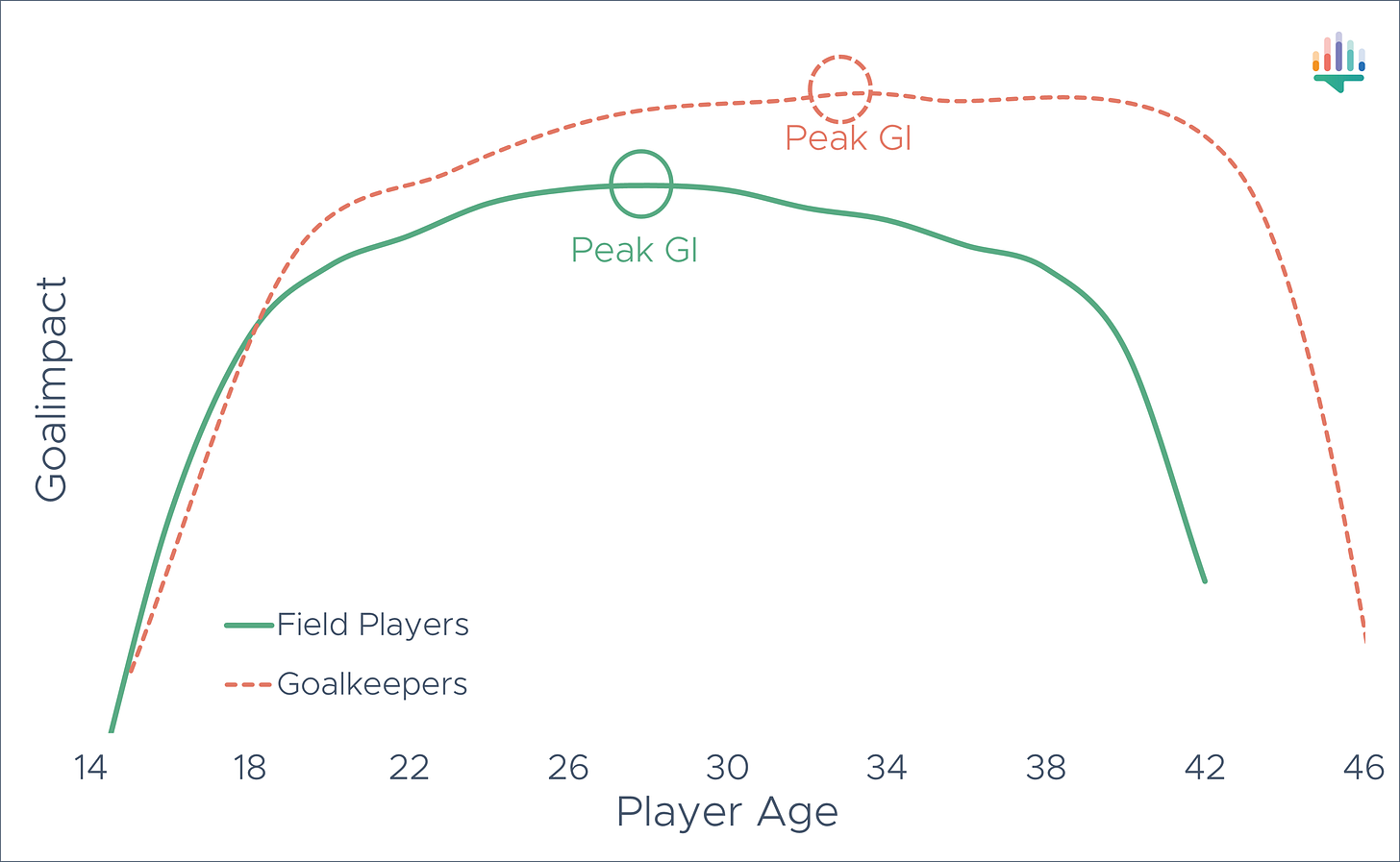
Goalkeepers are an extremely influential part of the game. An error by a goalie usually directly leads to conceding a goal. In contrast, field players' mistakes can often be corrected because of consecutive steps until a goal is conceived. Such position-dependent error impact creates a higher incentive for goalkeepers to minimize mistakes. Therefore a goalkeeper's impact might be more influenced by experience and less connected to pure physical abilities. As a consequence, Goalkeepers have their Goalimpact Peak at around age 33.
Furthermore, goalkeepers are not required to sprint, which, as we pointed out, might be a key factor for stronger performance decline in field players above 30. Consequently, it is no surprise that Goalkeepers age much slower according to the Goalimpact aging curve. After all, reflexes and jumping ability can probably be sustained to an older age. Their level of performance remains relatively stable until the age of 42. It thus pays off for clubs to buy experienced goalkeepers because the return on investment on points is pronounced.
Regardless of the advice for signing old-but-gold goalkeepers, the most practical knowledge is covered in the aging curve regarding young talents.
Talents and a systemic bias
It is no secret that from a young age, talents are already scouted and signed by professional clubs. Yet there is a systemic bias and discrimination on-going. Players born at the end of a year (like myself) are dramatically underrepresented in almost all big leagues. This refers to the relative age bias. Why?
The aging curve shows that the biggest rise in Goalimpact occurs between the age of 14 to 16 (Note: Data for younger players are unavailable and/or reliable. Thus, the biggest difference per year occurs probably even before). Within these two years, however, the Goalimpact increases by more than 100. This extreme growth can explain the remarkable selection bias in youth football.
Assume you are born on the 31 of December and just turned 14, while your oldest teammate is turning 16 on the first of January (Remember; usually players with two years different belong to one age category). You are basically two years younger than your oldest teammate - and hence your physical appearance is much less mature. Talents born in the beginning of a year thus dominate mainly by their physical superiority.
The relative age difference (14.7 % younger in the extreme case mentioned above) and the resulting selection bias is well reported in football. For example, in 2018 140 players in the 1. and 2. Bundesliga were born in January and only 46 in December. This asymmetry is permanent across all years - not just a particular season's stat. Roughly 75 % of German youth players in professional clubs are born in the first half of a year (even though there are more births in the last half of a year.) Interestingly this bias still occurs when a club is aware of the relative age effect.
The selection bias can also be analyzed in a Goalimpact scatter plot (see below). Among the youth players (blue area) we see three thick vertical lines (yellow marked). These players are born in the first half of the year and are overrepresented in youth teams of professional football clubs because they have a physical advantage. Once they are fully mature and grown, the advantage disappears, and so do the vertical lines (from age 20 on). We conclude that many players, favored by their age, don't make it to senior football. But given that many players born in January to June drop out later, many resources are wasted on players with a bad prognosis to make it to senior football anyway.
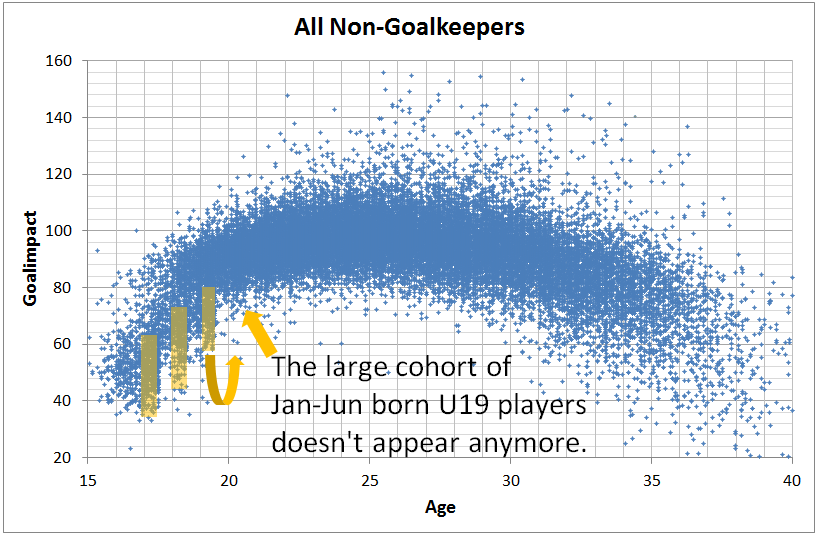
We, therefore, propose to select the players by prognosis rather than current physical development because this will improve resource allocation. One way of doing so would be selecting players by PeakGI.
But there are already other approaches to cope with the Bias.
Belgium - The Innovator
A way to improve the selection of talents is to organize young players according to their maturity status (commonly referred to as 'bio-banding') instead of their birth date. Thereby extreme physical discrepancies are reduced and hence smaller (=younger) players would not be dominated entirely by the physical superior.
In addition, a 'rotating deadline' could be implemented to change age distribution in youth teams. If the deadline from one youth class to the next was shifted by three months, sooner or later, everyone would be among the oldest.
The Belgic football association executes both ideas. The consequence: Belgium displays almost a normal distribution of player age in their professional league and national team. Such “fair” distribution is empirical evidence that Belgium develops and includes more talents, especially those born late in the year. The inclusive approach might be the crucial explanation for why the Belgian national team, even though Belgium has a small population (11-12 million people), is second in the FIFA Football Ranking list (only behind Brazil). Considering European giants in football, England, Spain, France, Germany, and Italy is quite miraculous.
Goalimpact supports such data and assumes that Belgium is Number 4 in the World (despite their poor performance at WC 2022).
Why Haaland is not an "average player"
Despite the benefits of an average aging factor, there is one major drawback:
The general is not the Particular.
Meaning: A specific player might deviate strongly from the expected development.
There is a relatively large standard deviation, especially for young players, where the number of minutes (sample size) is relatively low. To improve the prediction of player development, thus other (non-Goalimpact) players' characteristics could be considered.
We already mentioned how speed might particularly impact older players. However, additional factors might be essential for predicting the overperformance of young talents. Such early indicators might include the obsessive desire to improve, high intelligence, staying healthy, and good coaches (Klopp, Guardiola) who ideally nurture a player's development. Those elements create a black box, which introduces uncertainty in a player's development. Consequently, giving a precise prediction is impossible. However, noticing such indicators enables football managers to anticipate potential overperformers intelligently.
Erling Haaland, for example, is a pretty straightforward model of how the predicted Goalimpact Performance can be exceeded. There is no doubt that he is an extremely obsessed and hard worker. Whether it is mental improvement in the form of meditation or taking extreme care of nutrition, Haaland tries to have the edge in every domain. He has a passion for becoming the very best. It is thus no surprise that he overperforms the average development. Given that he stays healthy, he will become one of the best and better than we predict.
This is already happening with Haaland. He has overperformed Goalimpact constantly for the last three years. The initial Peak prediction was 140 and is not Especially the psychological aspects can be well investigated with personality tools such as the Big Five OCEAN Model, which measures a person's basic motivational and character traits.
Nevertheless, just using the aging curve can tremendously improve the scouting and development of young players. Just eliminating the relative age bias should be reason enough to implement a systematic and strategic way to deal with young players.
Who knows how many world-class talents we discard?
What is Goalimpact?
Goalimpact measures the influence of a player on the goal difference.
It is thus objective player rating system and a risk management tool for signing football players.
See more about Goalimpact here or call us online right away to explore how we can help your club!
This is very interesting. Nice Post.
D.W.